报告题目:Exploring Trustworthy Foundation Models under Imperfect Data
报告时间:2024年6月6日16:00-16:50
报告地点:新葡萄8883官网AMG大楼B404会议室
报告人:韩波
报告人国籍:中国
报告人单位:香港浸会大学
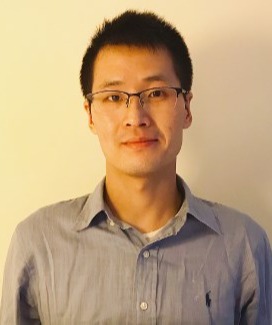
报告人简介:Bo Han is an Assistant Professor in Machine Learning at Hong Kong Baptist University and a BAIHO Visiting Scientist at RIKEN AIP, where his research focuses on machine learning, deep learning, foundation models and their applications. He was a Visiting Research Scholar at MBZUAI MLD, a Visiting Faculty Researcher at Microsoft Research and Alibaba DAMO Academy, and a Postdoc Fellow at RIKEN AIP. He has co-authored two machine learning monographs by MIT Press and Springer Nature. He has served as Senior Area Chair of NeurIPS, and Area Chairs of NeurIPS, ICML, ICLR, UAI and AISTATS. He has also served as Action Editors of MLJ, TMLR, JAIR and IEEE TNNLS, and Editorial Board Members of JMLR and MLJ. He received Outstanding Paper Award at NeurIPS, Notable Area Chair at NeurIPS, Outstanding Area Chair at ICLR, and Outstanding Associate Editor at IEEE TNNLS. He received the RGC Early CAREER Scheme, NSFC General Program, IJCAI Early Career Spotlight, RIKEN BAIHO Award, Dean's Award for Outstanding Achievement, Microsoft Research StarTrack Program, and Faculty Research Awards from ByteDance, Baidu, Alibaba and Tencent.
报告摘要:In the current landscape of machine learning, it is crucial to build trustworthy foundation models that can operate under imperfect conditions, since most real-world data, such as unexpected inputs, image artifacts, and adversarial inputs, are easily noisy. These models need to possess human-like capabilities to learn and reason in uncertainty. In this talk, I will focus on three recent research advancements, each shedding light on the reliability, robustness, and safety in this field. Specifically, the reliability will be explored through the enhancement of vision-language models by introducing negative labels, which effectively detect out-of-distribution samples. Meanwhile, robustness will be explored through our investigation into image interpolation using diffusion models, addressing the challenge of information loss to ensure consistency and quality of generated content. Then, safety will be highlighted by our study on hypnotizing large language models, DeepInception, which leverages the creation of a novel nested scenario to induce adaptive jailbreak behaviors, revealing vulnerabilities during interactive model engagement. Furthermore, l will introduce the newly established Trustworthy Machine Learning and Reasoning (TMLR) Group at Hong Kong Baptist University.
邀请人:杜博、王增茂
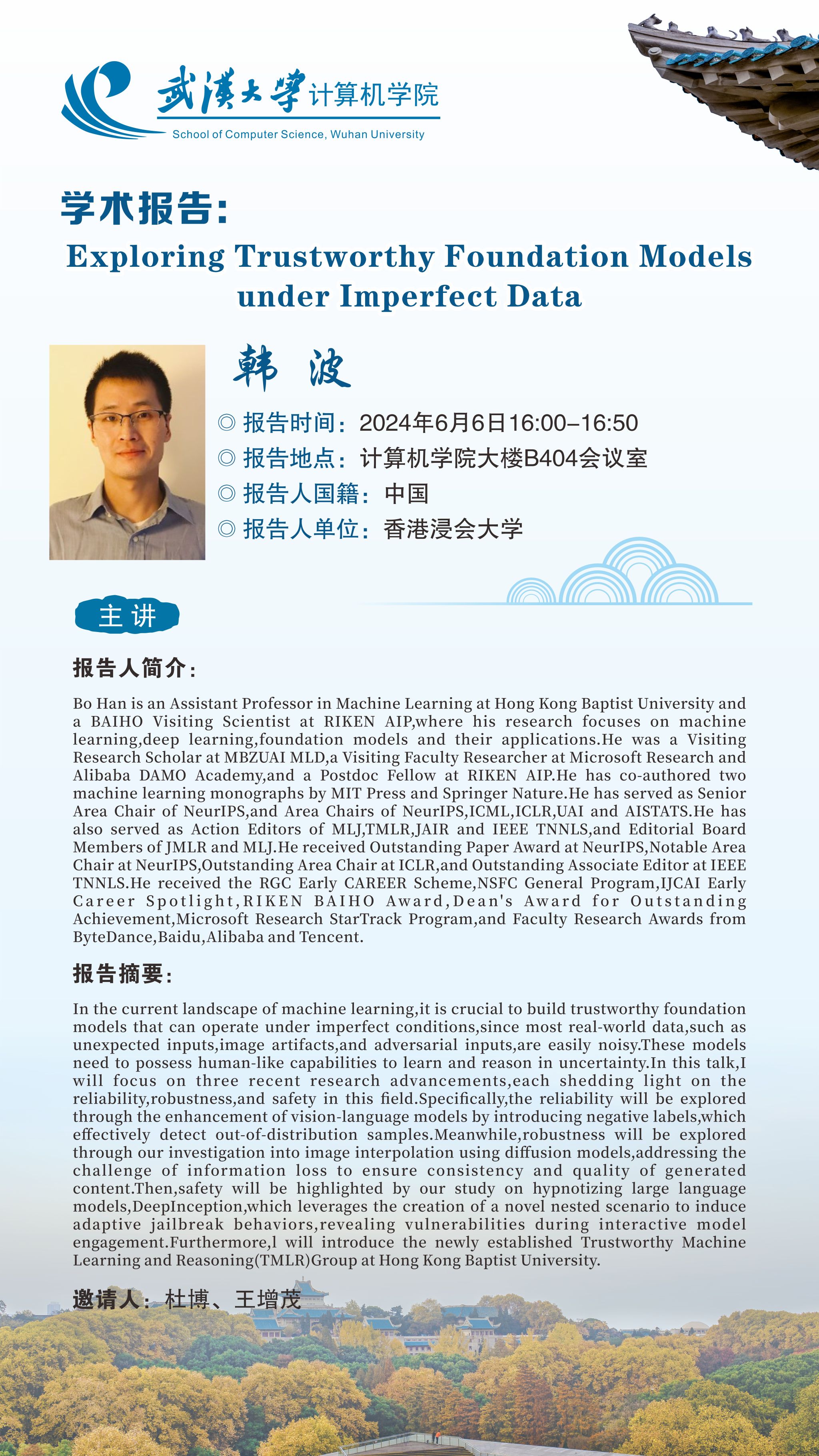