报告题目:Towards Graph-level Anomaly Detection via Deep Evolutionary Mapping
报告时间:2023年12月12日上午10:00
报告地点:新葡萄8883官网AMG大楼B404会议室
报告人:吴佳
报告人国籍:中国
报告人单位:麦考瑞大学
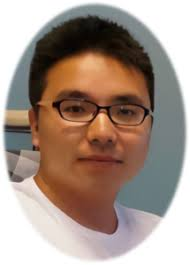
报告人简介:吴佳,澳大利亚麦考瑞大学人工智能中心研究主管(Research Director) 、国际数据挖掘顶级期刊ACM Transactions on Knowledge Discovery Data(TKDD)副主编。2019 Heidelberg Laureate Forum Fellowship – 澳洲科学院 (Australian Academy of Science)。澳大利亚麦考瑞大学新葡萄8883官网AMG教授、副院长分管博士研究生。主要研究领域为数据挖掘、机器学习、人工智能,及其在商业、工业、生物信息学、医疗信息学等领域的应用。迄今,在国际学术期刊和会议上共发表论文100多篇, 包括IEEE TPAMI、IEEE TKDE、IEEE TNNLS、IEEE TCYB、ACM TKDD、NeurIPS、WWW、ACM KDD、IEEE ICDM、ACM WSDM、IJCAI、AAAI、ACM CIKM等。指导学生获得2022年顶级信息检索领大会ACM CIKM最佳论文奖Runner-Up、2021年顶级数据挖掘大会IEEE ICDM最佳学生论文奖、2018顶级国际数据挖掘大会SIAM SDM最佳论文奖-Applied Data Science Track、2017顶级国际神经网络大会IEEE IJCNN最佳学生论文奖。
报告摘要:Graph-level anomaly detection aims at capturing anomalous individual graphs in a graph set. Due to its significance in various real-world application fields, e.g., identifying rare molecules in chemistry and detecting potential frauds in online social networks, graph-level anomaly detection has received great attention recently. Although deep graph representation learning shows effectiveness in fusing high-level representations and capturing characters of individual graphs, most of the existing works are defective in graph-level anomaly detection because of their limited capability in exploring information across graphs, the imbalanced data distribution of anomalies, and low interpretability of the black-box graph neural networks (GNNs). To overcome these limitations, we propose a novel deep evolutionary graph mapping framework named GmapAD, which can adaptively map each graph into a new feature space based on its similarity to a set of representative nodes chosen from the graph set. Through our extensive experiments on nine real-world datasets, we demonstrate that our method has achieved statistically significant improvements compared to the state of the art in terms of precision, recall, F1 score, and AUC.
邀请人:杜博